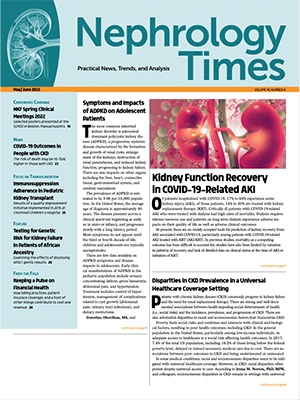
Following solid-organ transplantation, the primary aim of care is preventing allosensitization. Despite potent immunosuppression, nonadherence often disrupts treatment, resulting in rejection. Among recipients of kidney transplant, the strongest predictors of allograft failure are nonadherence and subsequent antibody or T-cell-mediated rejection (AMR and TCMR, respectively).
Adolescent and young adult kidney transplant recipients are disproportionally affected by suboptimal adherence, due, in part, to normal development changes affecting adherence behaviors (brain development, independence, risk taking, and peer influence). As a result, transplant recipients in that age group face rates of allograft failure that are three times those of older recipients, resulting in a need to return to dialysis, and contributing to a 25-year decrease in their life expectancy, as well as worsened quality of life and much higher cost.
Results of previous clinical trials have demonstrated that adherence barriers are amenable to behavioral interventions, and provider-delivered, systems-based, multicomponent interventions have had substantial and durable effects. However, according to David K. Hooper, MD, and colleagues, there are few data available on whether improved adherence results in allograft rejection.
The researchers reported results of a quality improvement initiative implemented in 2015 at Cincinnati Children’s Hospital aimed at decreasing late acute rejection in kidney transplant recipients by applying evidence-based adherence strategies as standard care. The strategies directly addressed barriers to adherence and correlated highly with adherence behaviors. Results of the initiative were reported in the American Journal of Kidney Diseases [2022;79(3):335-346].
The initiative included kidney transplants who were receiving care at Cincinnati Children’s Hospital ≥1 year after transplant and who were taking one or more immunosuppressive medications from 2014 through 2017. Over a 14 month period, interventions collectively called MAPS (Medication Adherence Promotion System) were implemented: (1) adherence promotion training for clinical staff; (2) electronic health report-supported adherence risk screening; (3) systematic assessment of medication adherence barriers; (4) designation of specific staff to partner with patients to address adherence barriers; (5) patient-centered shared decision-making to develop action plans; (6) follow-up evaluation to address progress and adapt action plans if necessary; and (7) optional electronic medication adherence monitoring.
The primary outcome measure was biopsy-proven late acute rejection, acute TCMR grade 1a or higher or AMR according to the Banff 2013 criteria. Process measures were conducted to assess barriers, identify barriers, and perform interventions. Secondary outcomes/balancing measures were de novo donor-specific antibodies (DSA), biopsy rate, and rejections per biopsy.
Patient-days between acute rejections as well as monthly rejections per 100 patient-months before and after implementation of the program were evaluated using time series analysis with statistical process control. Multivariable analyses were performed to control for known rejection risk factors including changes in treatment and case mix.
Over the 4-year study-period, the researchers assessed 121,133 active patient-days in 130 patients. A total of 72 patients met inclusion criteria at the beginning of the observation period. During the study, 58 patients entered the cohort (49 new transplants and nine transfers). Forty-three left the cohort. Reasons for leaving the cohort were transferred care (n=33), allograft failure (n=4), loss to follow-up (n=3), death (n=2), or malignancy (n=1). All of the allograft failures occurred in the pre-MAPS period.
There were 51 rejections (34 prior to MAPS vs 17 after MAPS). Twenty-four patients had one rejection, four patients had two rejections, five patients had three rejections, and one patient had four rejections. Of all patients-days of observation among patients with multiple rejections, the proportion occurring in the pre-MAPS and post-MAPS periods were similar (59.9% vs 48.1%), which was comparable to the entire sample.
During the 26-month baseline and implementation period, there was a median of 1700 (upper control limit [UCL], 7939) patient-days between rejection episodes. Prior to implementation of MAPS, the monthly rejection rate was 1.61 rejections per 100 patient-months.
There were four rejection episodes in the 5 weeks following implementation of MAPS. After April 7, 2016, the researchers observed 11,658 patient-days between rejection episodes above the previous UCL of 7939. Following implementation of MAPS, the average monthly late acute rejection rate fell from 1.61 to 0.88 rejections per 100 patient-months. All but three of the 22 monthly data points after implementation of MAPS fell below the previous monthly average rejection rate, including the final 12 consecutive months indicating special cause on the U-chart. There were an estimated 14 fewer rejections in the 22-month post-implementation period than predicted by the prior 2 years.
In the multivariable model, the post-MAPS treatment period was associated with a 50% reduction in the incidence of rejection (incidence rate ratio [IRR], 0.51; 95% confidence interval [CI], 0.28-0.94; P=.03). The final model included DSA history, repeat kidney transplant, donor type, race, prior rejection, human leucocyte antigen mismatch, and time since transplant. None of the covariates significantly violated the proportionality assumption.
Following adjustment for covariates in the multivariable model, the post-MAPS treatment period was associated with a 50% reduction in the incidence of rejection (IRR, 0.50; 95% CI, 0.27-0.91; P=.02). There was an association between DSA history and a >2-fold increase in incidence of rejection (IRR, 2.27; 95% CI, 1.12-4.57; P=.02). There was also an association between each year after transplant and a 13% decreased incidence of rejection (IRR, 0.87; 95% CI, 0.77-0.97; P=.02).
There were no significant differences in the rate of kidney biopsies per 100 patient-months before and after implementation of MAPS (3.17 vs 2.91; P=.5). There was a significant decrease in the rate of biopsies positive for rejection (50% to 30%; P=.03). A total of 13 patients developed de novo DSA during the pre-MAPS period compared with seven patients in the post-MAPS period (P=.3). There were no significant differences in the severity and type of rejection before and after implementation of MAPS (P=.8).
Limitations to the findings cited by the authors included lack of direct measuring of adherence, the single-center study design, and the need to make inferences of causality.
In summary, the researchers said, “We adapted evidence-based adherence interventions and reliably implemented them at the point of care with clinical staff supported by the EHR. This multimodal, provider-delivered system to identify and mitigate patient and caregiver barriers to taking immunosuppression was associated with a 50% rejection in late allograft rejection. MAPS has become the standard of care in our clinic and should be tested in other settings.”
Takeaway Points
- Among pediatric kidney transplant recipients, suboptimal adherence to immunosuppression medications is associated with high risk of allograft rejection.
- Clinicians at Cincinnati Children’s Hospital implemented a program to help patients identify barriers to medication adherence and work with clinicians to manage those barriers.
- Following full implementation of the system, the rate of acute allograft rejection decreased by nearly half.