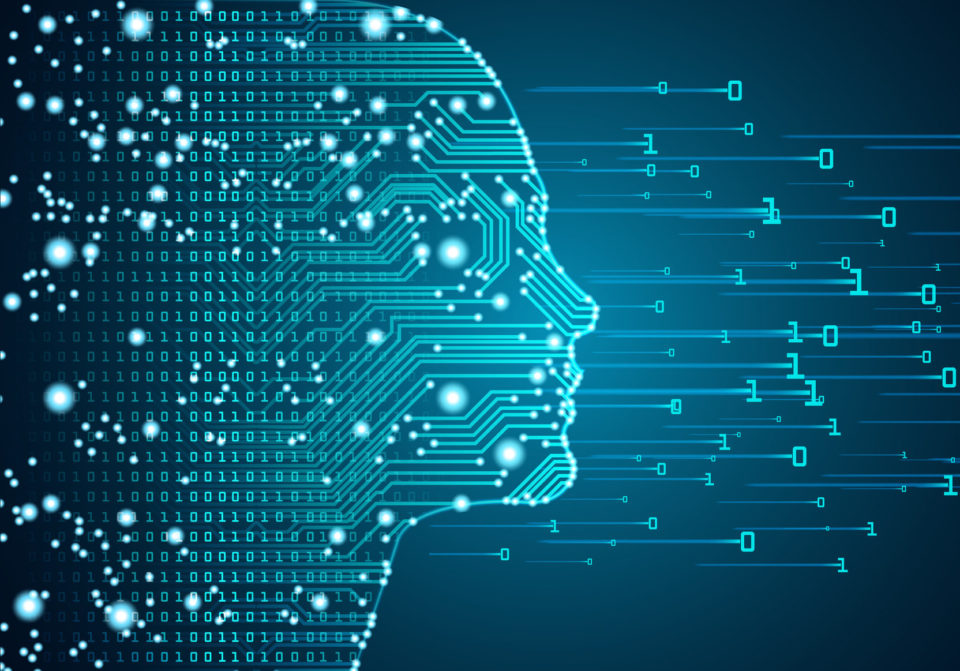
A presentation during The American College of Rheumatology/Association of Rheumatology Professionals Annual Meeting discussed the current status of the use of artificial intelligence (AI) and big data in various rheumatic and musculoskeletal diseases (RMDs).
Big data are large datasets that may be too complicated to be deciphered with traditional data-processing software, the study authors described. In some instances, AI could be beneficial here.
“The current use of these concepts in publications related to rheumatic and musculoskeletal diseases (RMDs) is unknown,” the researchers posited. “Therefore, the aim of this literature review was to assess the current use of big data and AI in the field of RMDs.”
The literature review included a search of PubMed MEDLINE with key words related to big data, AI, and RMDs. Only reports published in English were included. For comparison, the authors also searched for reports outside the scope of RMDs. The researchers evaluated how much data were analyzed as well as what data sources and statistical methods were used (traditional, AI, or both).
AI Use in RMDs and non-RMDs
Among 567 RMD-related articles discovered in the search, 55 RMD articles were included in the analysis and compared to 55 articles in other medical fields. Most of the RMD articles (72%) were published between 2013 and 2018, for a mean publication year of 2014; all of the articles in other medical fields were published in 2018 or 2019. The majority of the RMD articles pertained to inflammatory joint diseases (n = 22, 40%) or osteoarthritis (n = 16, 29%), with the three most highly represented diseases being knee osteoarthritis (n = 13, 24%), rheumatoid arthritis (n = 12, 22%), and post-menopausal osteoporosis (n = 6, 11%). Among the non-RMD articles, the most commonly discussed medical fields were oncology (n = 14, 25%), neurology (n = 8, 15%), infectious diseases (n = 6, 11%), ophthalmology (n = 5, 9%), and psychiatry (n = 5, 9%).
“Big data” was not specifically defined in most of the articles (RMDs, n = 2, 4%; and non-RMDs, n = 7, 13%). Among the RMD articles, the mean number of data points was 746 million (range 2,000–5 billion), compared to 9.1 billion (range 100,000–200 billion) among the non-RMD articles. Representation of data sources varied among the RMDs (clinical, 47%; biological, 15%; and radiological, 29%), but was more similar among the non-RMDs (clinical, 31%; biological, 31%; and imaging sources, 29%).
Traditional big data analysis were observed in 18% of RMDs and 15% of non-RMDs, and AI methods were observed in 82% and 85%, respectively. Nearly all of the AI papers discussed machine learning (RMDs, n = 44/45; non-RMDs, n = 47/47), the most common of which was artificial neural network (RMDs, n = 20/44; non-RMDs, n = 24/47).
The study authors concluded in their abstract, “Big data sources and types are varied within the field of RMDs, and methods used to analyze big data were heterogeneous. These findings informed a EULAR taskforce developing recommendations for big data in RMDs.”