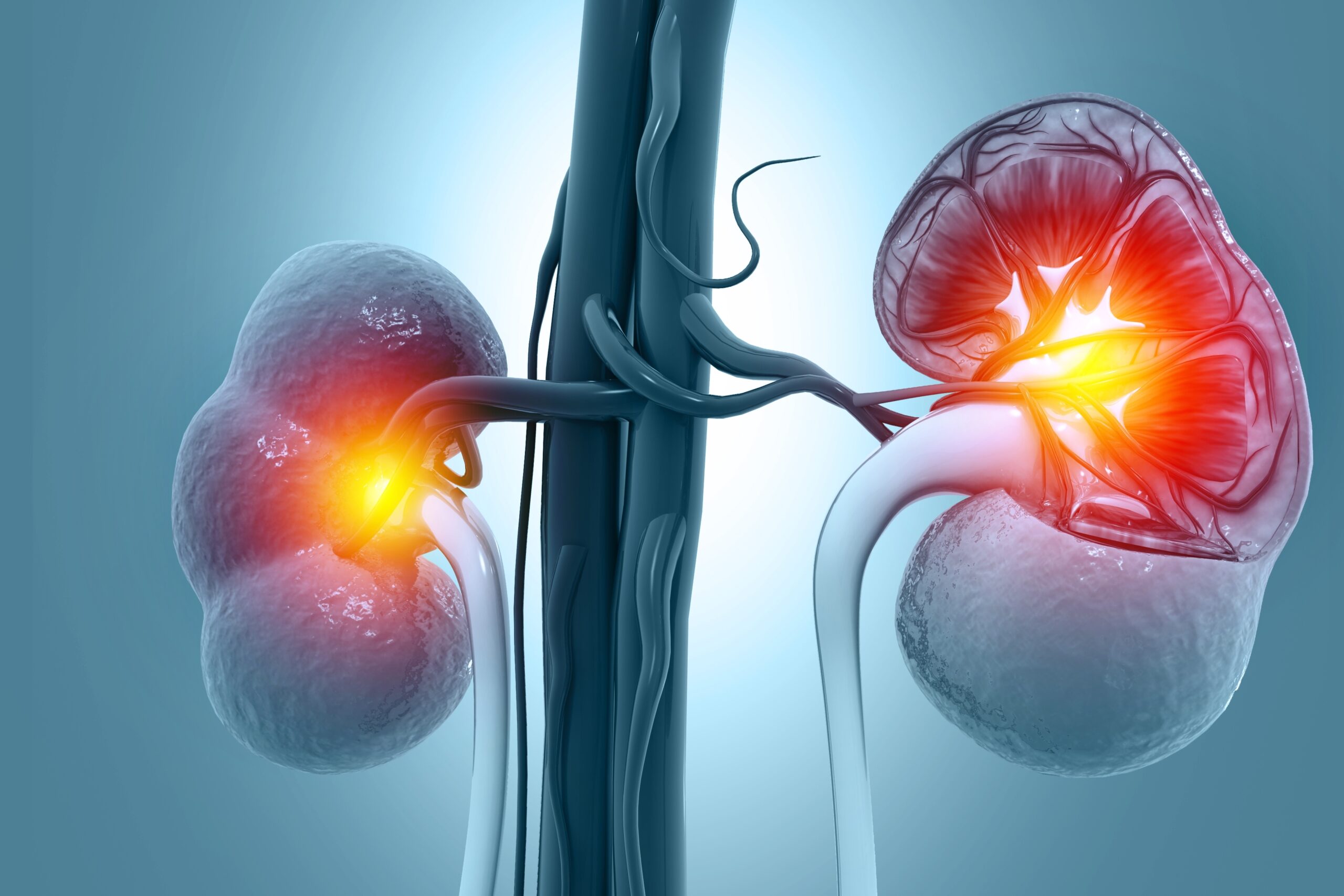
In a proof-of-concept study presented at the 61st European Renal Association Congress, Anibal Pedraza and others attempted to develop a machine learning classifier for 12 different classes of glomerulonephritis (GN) with convolutional neural networks and self-attention-based architectures.
Their dataset comprised 11,000 period acid-Schiff (PAS)-stained glomerular crops from 350 biopsies across four institutions. Each crop retained the diagnosis label from the 12 classes: (1) antibody-mediated GN; (2) anti-neutrophil cytoplasmic antibody; (3) C3-GN; (4) cryoglobulinemic GN (CryoGN); (5) dense deposit disease; (6) fibrillary; (7) infection-associated GN; (8) IgA GN; (9) membranoproliferative glomerulonephritis; (10) membranous; (11) proliferative GN with monoclonal immunoglobulin G deposits (PGNMID); and (12) systemic lupus erythematosus GN class IV. A 13th class, sclerotic, was created by stripping globally sclerotic glomerular crops from this diagnostic label.
Of the total dataset, 75% of samples were used for training, 15% for validation, and 10% for testing. A validation set comprising another 50 biopsies from an additional three centers yielded 2000 new crops. A classifier was trained and supervised for the 13 classes based on a collective of multiple transformer-based classification networks, including Swin-Transformer and ConvNext. Each network was trained under different circumstances, ensuring the system acquired a more global understanding versus relying on a sole method. To make the final decision, the system uses the prediction with the largest confidence threshold.
Metrics for classification performance were measured as precision, sensitivity, specificity, F1 score, and balanced accuracy. Balanced accuracy ranged from 0.4797 for CryoGN and 0.5949 for membranous. It was 0.6892 for the sclerotic class. Areas under the receiver operating characteristic curve were between 0.40 for PGNMID and 0.82 for membranous, and 0.81 for sclerotic.
Such complex classification generally requires immunostains, electron microscopy, and clinical data, but this study creates a baseline for classification. In sum, the authors wrote, “Our classification results even on single PAS glomerular crops appear promising. Combined with our automatic glomerular segmentation models, we could rapidly expand the training cohorts’ sizes and even add more classes of GN.”
Source: Pedraza A, Becker J, Altini N, et al. Machine learning glomerulonephritis diagnosis on single glomeruli. Abstract #3070. Presented at the 61st European Renal Association Congress; May 23-26, 2024; Stockholm, Sweden.