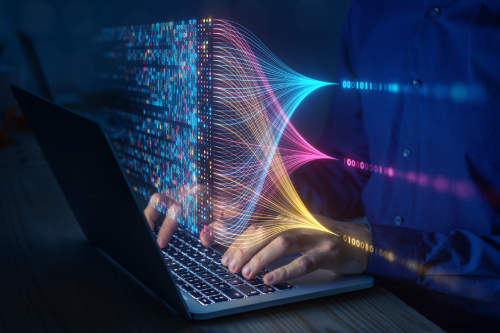
Integrating clinical, dual-energy spectral computed tomography (DESCT), and radiomics features may “enhance the prediction” of mutated KRAS in patients with the lung adenocarcinoma subtype of non-small cell lung cancer (NSCLC), according to a recent study.
The study was published in Translational Lung Cancer Research by a team of researchers from the National Cancer Center/National Clinical Research Center for Cancer/Cancer Hospital, Chinese Academy of Medical Sciences and Peking Union Medical College, in Beijing.
The lung adenocarcinoma subtype of NSCLC is “often associated with genetic alterations,” such as the KRAS mutation, which is “particularly challenging to treat due to resistance to targeted therapies,” the researchers explained. This led the researchers to develop a predictive model for the KRAS mutation in these patients by integrating clinical, DESCT, and radiomics features.
The study investigators retrospectively enrolled 172 patients with lung adenocarcinoma and divided them into a developing cohort (n=120) and a validation cohort (n=52). The researchers extracted and analyzed clinical, DESCT, and radiomics features to construct four predictive models, including:
- Clinical features model
- DESCT features model
- Radiomics features model
- Combined clinical-DESCT-radiomics (C-S-R) model.
The study investigators evaluated the models’ performance by the receiver operating characteristic curves and developed a nomogram incorporating clinical, DESCT, radiomics features with R-score in the validation cohort.
Among the 172 patients in the study, 8.7% (15) had a KRAS mutation. The C-S-R model “demonstrated the best performance,” with an area under the curve (AUC) of 0.92 in the developing cohort and 0.87 in the validation cohort, according to the researchers. However, the C-S-R model “was not superior to radiomics model” (P=.28), but it was “significantly better” than DESCT model (P=.01).
Based on these findings, the study authors concluded that integrating these three features can “enhance the prediction of the KRAS mutation” in patients with lung adenocarcinoma.