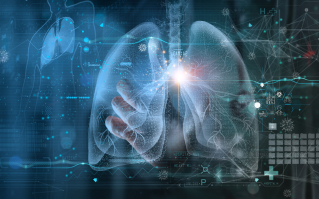
HistoTME, a novel weakly supervised deep learning approach, may be an effective way to characterize the tumor microenvironment (TME) molecular composition and predict responses to immune checkpoint inhibitors in patients with non-small cell lung cancer (NSCLC), according to a recent study.
Alex H. Chen, of the National Cancer Institute, and colleagues conducted the study and presented the results at the 2024 Society for Immunotherapy in Cancer Annual Meeting.
“With artificial intelligence, there is great potential to unlock TME-related information related to Hematoxylin and Eosin (H&E)-stained pathology slides, which are readily accessible through clinical routine,” Chen and colleagues explained.
It was important to conduct the research because immune checkpoint inhibitors have become “integral” to the treatment of NSCLC, but there is a “clinically unmet need for additional predictive biomarkers capturing tumor and microenvironmental factors” that are associated with responses to immune checkpoint inhibitors.
The team developed HistoTME to infer the TME molecular composition directly from histopathology images of NSCLC, and the model “learns to predict the gene expression levels of 30 cell type-specific TME signatures from H&E whole slide images.” The system was trained by using H&E images and matched bulk transcriptomics data from the TCGA-NSCLC cohort (N=865) and validated using an external cohort of CPTAC-NSCLC (N=333).
The researchers used TME signatures to characterize TME status as “inflamed” versus “desert” and to predict response to immune checkpoint inhibitors. They tested these methods retrospectively using survival outcomes from an external cohort of 292 patients with NSCLC who were treated with immune checkpoint inhibitor therapy.
The study showed that HistoTME “accurately predicts the expression” of 30 TME molecular signatures from H&E images, showing an average Pearson correlation of 0.50 with the ground truth. The researchers used HistoTME-predicted signatures to derive TME “inflamed” versus “desert” status, “improving prognostication” of patients receiving first-line immune checkpoint inhibitor treatment (P=.0012; hazard ratio [HR], = 0.52; 95% CI, 0.35-0.77). Patients who had PDL1 expression ≥50% also showed improved survival (P=.0059; HR, 0.62; 95% CI, 0.37- 1.04).
In an additional subgroup analysis, the study showed that the H&E inferred TME status is “predictive of overall survival outcomes of patients with absent PD-L1 (<1%; P=.08; HR, 0.4; 95% CI, 0.13- 1.18]), and low PD-L1 (1-49%; P=.009; HR, 0.44; 95% CI, 0.23-0.82). However, this was not the case in those with high PD-L1 (≥50%; P=.85; HR, 0.94; 95% CI, 0.48-1.84). In addition, the signatures “could be utilized to accurately predict response,” achieving an AUROC of 0.75 (95% CI, 0.61-0.88) for predicting responses following first-line immune checkpoint inhibitor treatment.
“Collectively, HistoTME presents an effective approach for characterizing the TME molecular composition and predicting [immune checkpoint inhibitor] response for NSCLC patients, complementing the current clinical biomarker, PD-L1 expression, and bringing us closer to personalized immuno-oncology,” the researcher concluded.
Reference
Chen A, Patkar S, Basnet A, et al. Predicting the tumor microenvironment molecular composition to assess immunotherapy efficacy in non-small cell lung cancer from digital histopathology images. Abstract #77. Presented at the 2024 Society for Immunotherapy in Cancer Annual Meeting, November 6-10, Houston, Texas.