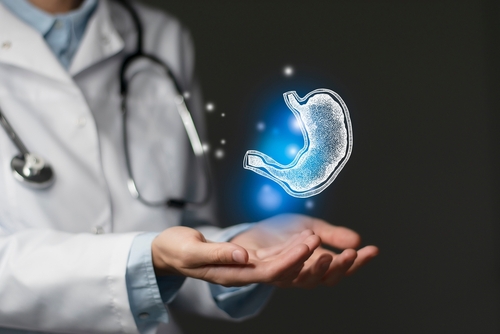
Neuroendocrine tumor (NET) diagnosis is often delayed due to misdiagnosis or nonspecific symptoms, resulting in the occurrence of metastases in patients.
To enhance the detection of NETs in patients, a recent study sought to develop and validate a multi-gene native elongating transcript sequencing (NETseq) ensemble classifier using peripheral blood RNA sequencing to identify NET-related biomarkers and determine treatment response in patients receiving 177Lu-DOTATATE peptide receptor radionuclide therapy (PRRT).
Blood samples were taken from a total of 178 patients with NETs and 73 healthy patients, which were then analyzed using RNA sequencing. A learning cohort of 59 PRRT-naïve patients with gastroenteropancreatic (GEP)-NETs and 38 healthy donors was formed to identify distinguishing gene features.
Five separate machine learning algorithms were trained and validated in the evaluation cohort (n=106), and the response to PRRT was measured in a PRRT cohort (n=46) and a PRRT response monitoring cohort (n=16).
The ensemble classifier determined NET from healthy patients samples with 100% accuracy in the learning cohort. In an evaluation cohort, the classifier demonstrated a 93% sensitivity rate (95% CI: 87.8%-98.03%) and a 91.4% specificity rate (95% CI: 82.1%-100%) for PRRT-naïve GEP-NETs (AUROC=95.4%). The classifier also demonstrated an >87.5% sensitivity rate across different tumor characteristics, outperforming serum Chromogranin A sensitivity (χ2=21.89, P=4.161e−6).
In the PRRT cohort, significantly lower NETseq prediction scores were seen after treatment with 177Lu-DOTATATE PRRT in comparison to non-responders. In an independent response monitoring cohort, paired samples (before PRRT and after the 2nd or 3rd cycle of PRRT) were analyzed.
The NETseq prediction score significantly decreased in partial responders (P=.002) and slightly reduced in those with stable disease (P=.068). The NETseq ensemble classifier identified PRRT-naïve GEP-NETs with ≥92% accuracy, and can play a potential role in early treatment response monitoring in the PRRT setting.
This blood-based, non-invasive classifier can serve as an optional tool for conventional methods in the detection and treatment response of patients with NETs.