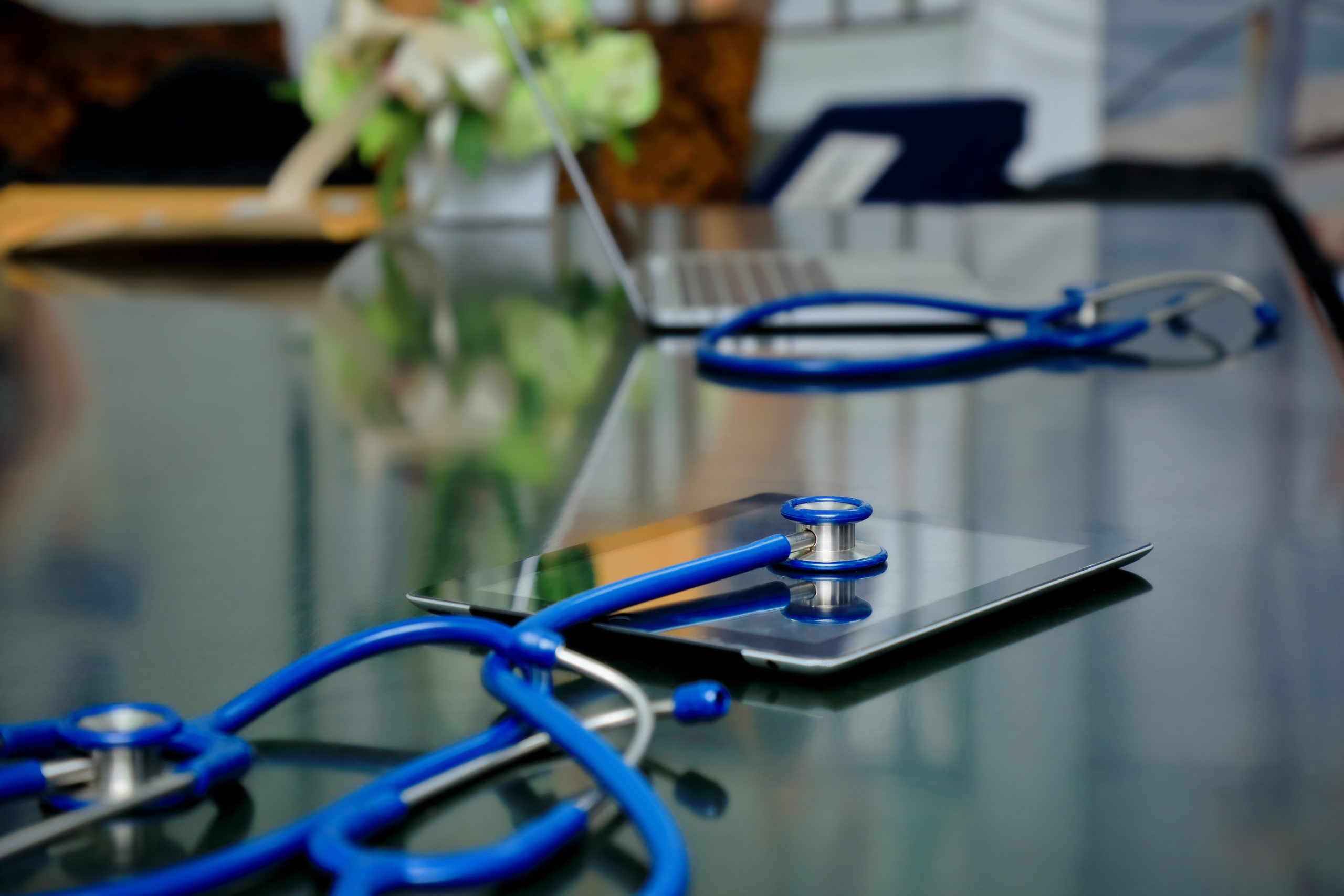
In a recent article in the Journal of Medical Economics, researchers evaluated the cost-effectiveness of implementing a machine learning risk prediction algorithm to identify patients with undiagnosed atrial fibrillation (AF) in primary care. According to the lead author Nathan R. Hill, “compared with routine care only, it is cost-effective to target individuals at high risk of undiagnosed AF through an AF risk prediction algorithm [to determine] who should then undergo diagnostic testing.”
The researchers utilized data from the PULsE-AI (prediction of undiagnosed atrial fibrillation using a machine learning algorithm) trial, which was conducted across 6 general practice centers in England from June 2019 to February 2021. Hybrid decision tree and Markov disease models were used to evaluate the cost-effectiveness of the risk algorithm. Outcomes included number of patients screened, number of AF cases identified, mean total and incremental costs, quality-adjusted life-years (QALYS), and the incremental cost-effectiveness ratio (ICER).
Cost Efficacy and Outcomes of the PULsE-AI Algorithm
According to the authors, the screening strategy resulted in an estimated 45,483 new diagnoses of AF across the high-risk population of 3.3 million individuals in the UK, as well as an estimated 14,004 lifetime diagnoses compared to routine care alone.
The individual-level costs for high-risk patients who underwent the study’s screening strategy were estimated to be £1,985, compared to £1,888 for patients receiving routine care only. Additionally, the screening strategy was attributed to a cost increase of £322 million and an increase of 81,000 QALYs.
Ultimately, the authors reported that the atrial fibrillation screening strategy was cost-effective compared to routine care “only at an accepted ICER threshold of £120,000 per QALY gained, with an ICER of £3,994/QALY.” They suggested that their study “highlights the potential value to both patients and the healthcare system in AI-based methods to support screening for AF.”
Find More Research at the Atrial Fibrillation Knowledge Hub